When products think: navigating the AI product shift
#225 - Apr.2025
What if the way we've interacted with computers for the last 60 years radically changes in a short period of time?
How do we build customer trust when apps can make decisions on their own without direct commands?
I've been reflecting on these questions. I'm sure many product and design teams are also wondering about the size of this change and what it means for our work.
Things are changing very fast.
AI is not only opening the doors for a new type of innovation, but is reshaping the way how we design products.
We are entering a new era in digital product design. A creative revolution that will rewards people who are curious to explore, iterate and discover new possibilities through technology.
A revolution that will make us rethink how product should be built and how they should evolve.
It might sound like a radical change. Indeed, is likely to be one. Yet, at the foundation, there is something about design that remains: we will continue to design for people.
Technologies will evolve. Our goal as designers and product leaders is to continue addressing people's needs and expectations. Empathy will remain as our north star for design decisions that are becoming more complex and ambiguous.
But many other things will change...
A new interaction paradigm
In Jakob Nielsen's words, AI represents "the third user-interface paradigm in the history of computing — the first new interaction model in more than 60 years."
A paradigm shift.
All these years we have been used to give commands to computers. Each command has a deterministic output.
Traditional digital products succeeded through predictability.
Users learn how to use a product and expect a result. You click on this button, and an action will happen.
For the last decades, predictability has been the key driver for building trust with customers. But what happens when the interaction changes from a command to an intent?
AI products operate differently.
In all GenAI tools available today we specify an outcome, not the how.
This fundamentally changes how we approach product development. We'll need to design for a world where users tell computers what they want, not how to do it.
Let me give you an example. Think about user acceptance tests:
- How do you approach a test where you are not evaluating the process execution but actually the outcomes?
- What is the criteria for 'Done' when working with a model that evolves over time?
- How do you design mechanisms that create consistent outcomes? Is consistency even necessary? How does this affect customer trust?
When a system's decisions become more complex and less transparent, how do we keep this trust? As Nielsen observes, "when users don't know how something was done, it can be harder for them to identify or correct the problem."
This is a new design challenge. And it's not a small one.
Traditional vs. AI Product Management
Think about the entire range of activities and responsibilities of a product leader. From discovery, prioritization, building and delivery. This paradigm shift adds new dimensions to each of these phases:
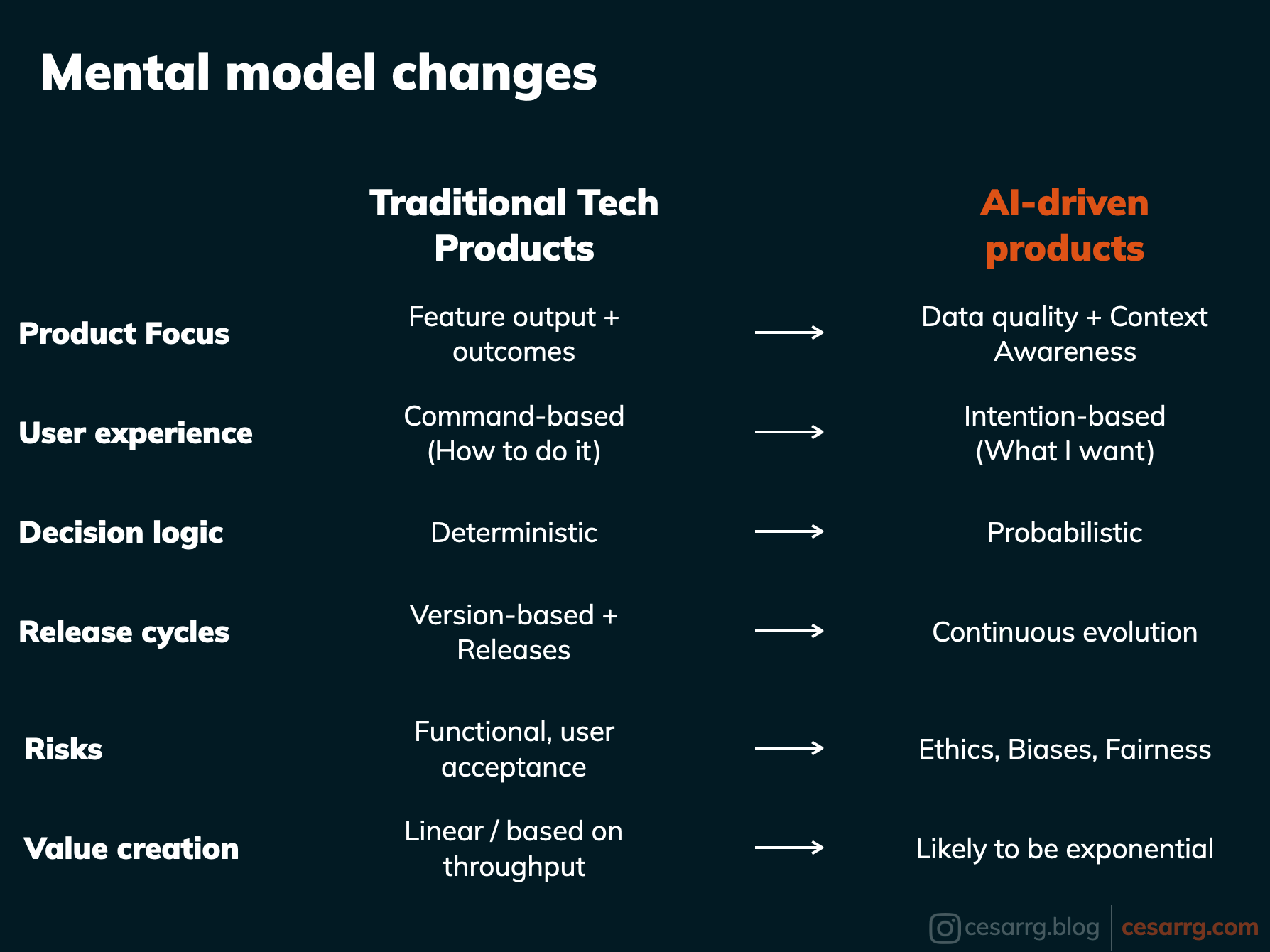
Starting with Product Focus. Traditional product management centers on outcome-driven features and UX. With AI products, we must equally prioritize features, data quality, and model performance. Your data strategy becomes as important as—or even more important than—your feature strategy.
User Experience. As customers shift to intention-based interactions with software, we need new tools and methods to manage their experiences. Not only on how the system reacts to such requests, but how to educate users on the best way to share their intent with the system.
Decision Logic. Traditional products deliver deterministic results — the same input always produces the same output. AI products operate probabilistically. Results may vary and improve over time as the model learns. We need to design the guardrails to handle customer expectations, anticipating wrong outputs due to hallucinations and providing transparency to customers on how decisions were made.
Release Cycles. Traditional products follow defined release cycles with clear versioning. AI products often use continuous deployment models where the system is constantly learning and evolving. While model's versioning continues to be applicable, we require new monitoring, validation, and rollback strategies for systems that are in constant evolution.
Risks. Traditional product management anticipate all sorts of risks, from purely functional ones (will the product serve its purpose?) to feasibility, usability and business risks. With AI, risks that were often neglected become foundational. Risks on how we remove biases, how we promote fairness and how we incorporate design principles over ethics will become the new norm.
Value Creation. Traditional products typically grow linearly based on feature addition. AI products can improve exponentially as adoption increases due to these data network effects. This creates a different scaling dynamic, where user adoption curves can take us by surprise. While scalability has been a core part of system design, now we are playing in new fields of scaling.
Discover actionable insights and expert tips to transform how you approach innovation, technology, and creativity. Practical tools you can apply immediately. Yes, they are free!
Measuring success
Our measurement tools must evolve with our products.
While traditional products might track clicks, conversion rates, and task completion, AI products require different success metrics:
- How often users need to refine their prompts to get what they want?
- What is the level of confidence from users on the outputs?
- How are we iterating over customer feedback to increase alignment?
Pay special attention to moments of delight versus frustration – understanding when variability creates value versus confusion.
Success in AI products may look different too.
Is less about task completion and more about goal achievement. Jobs-to-be-done will take the front seat to guide product teams towards the right success metrics.
We are entering a new era of product design and development. An exciting one!
The opportunities are huge if you embrace these changes with an open mind and, sometimes, breaking the traditional way of doing things.
A time to experiment, learn and iterate.
¡Saludos!